Comprehensive ML Pipelines
Automate the entire Machine Learning process, from feature extraction to model deployment, in Life Sciences.
Accessible to All
Empower non-experts by eliminating the need for advanced technical knowledge in AI and ML, ensuring equal opportunities globally.
Versatility in Applications
Apply BioAutoML to various types of structured and unstructured biological data, including DNA, RNA, and protein sequences, to address complex challenges.
Data-Centric Focus
Prioritize data quality and representation, ensuring robust AI model performance across diverse biological scenarios.
About
Find Out More About Us
We have been working on developing solutions to democratize AI, specifically Machine Learning (ML) in biology. So far, our studies have generated solutions that can be applied in the study of biological data, having a high potential to significantly reduce the experience required to use AI/ML pipelines, helping researchers in combating various problems, such as diseases that directly impact people's lives, mainly in low- and middle-income countries, giving biologists and other stakeholders, an opportunity for widespread use of these techniques.
BioAutoML
BioAutoML (a set of solutions) automates the entire ML pipeline, making it accessible to non-experts. It consists of two main components, each divided into four modules: (1) Automated Feature Engineering, which includes feature extraction and selection, and (2) Meta-Learning, which handles algorithm recommendation and hyperparameter tuning. BioAutoML can automatically extract features from diverse biological data, recommend algorithms, and fine-tune them for both binary and multiclass classification tasks. Our solutions operate without the need for specialized human intervention — all it requires is a training dataset to run a complete ML experiment. By simplifying these tasks, BioAutoML significantly lowers the barriers for non-experts to apply advanced ML techniques to biological data, advancing the democratization of AI in life sciences.
Publications
Our contributions have resulted in awards and published scientific articles, showcasing our commitment to advancing AI in biology - See FAQ.
Awards and Recognitions
BioAutoML has been recognized globally for its innovation and impact
Access to our solutions and articles
Awards and Recognitions
Stars - All Solutions
Citations - All Articles
Google Latin America Research Awards (LARA)
BioAutoML was selected among the 24 most promising ideas in Latin America, from a base of 700 submissions.
Santander X Brazil Award
BioAutoML was selected among the top 10 university projects (from over 200 entries) in Brazil in the national innovation competition promoted by Banco Santander.
Global Undergraduate Awards
BioPrediction (Bruno, André, and Robson) was awarded as the best undergraduate project in the world in computer science by the Global Undergraduate Awards 2024, marking the first time this award in the field has been given to Latin America.
Young Bioinformatics Award
BioAutoML received an honorable mention from the Young Bioinformatics Award 2024, being chosen among the best theses in Bioinformatics and Computational Biology in Brazil.
Artur Ziviani Thesis Award (SBCAS)
BioAutoML project received third place in the Artur Ziviani Thesis Award (SBCAS), being chosen among the best theses in computing applied to health in Brazil, 2024.
Global South Network - AI4PEP
AutoAI-Pandemics was selected as one of the most promising proposals (a total of 221 proposals from 47 countries) in a global competition.
Acceleration Program by ACE Cortex
BioAutoML received an intensive acceleration program by ACE Cortex (one of the largest in Latin America), focusing on innovation, scalability, and business development, as part of the Santander X Brazil Award.
Prototypes for Humanity - COP28-Dubai
Project selected (BioPrediction) to participate in Prototypes for Humanity 2023, during COP28-Dubai, chosen from 3000 entries, from more than 100 countries, standing out among the 100 best.
FEMS Research & Training Grant/Award
Federation of European Microbiological Societies (FEMS)
Helmholtz Visiting Researcher Grant/Award
Helmholtz Information & Data Science Academy (HIDA)
Falling Walls Lab Brazil
Finalists (Ideas Contest - Top 15 of 82), Falling Walls Lab Brazil 2022, DWIH São Paulo, Falling Walls Foundation, DAAD, The German Center for Research and Innovation.
Editor's Choice Article, Entropy
Recognized by the journal's Academic Editor as an exceptional contribution to the field. The article is featured in Entropy's special edition dedicated to showcasing groundbreaking research.
Scientific Initiation Competition (SBCAS)
Second place (BioPrediction) in the Scientific Initiation Competition (SBCAS), being chosen among the best works in computing applied to health in Brazil, 2024.
Our Solutions
Explore Our Innovative Tools
Empowering researchers with cutting-edge tools in Life Sciences and beyond.
BioAutoML-FAST
Empowering Breakthroughs in Life Sciences with End-to-End Machine Learning - Launching soon!
BioPrediction-PPI
Democratizing Machine Learning in the Study of Molecular Interactions - Launching soon!
Case Studies
Success Stories from Our Solutions
Explore how our solutions have made contributions to Life Sciences.
Anticancer Peptides
Utilization of advanced machine learning models to predict and analyze peptide sequences with potential anticancer properties
Long Non-Coding RNAs (lncRNAs)
Advanced classification and analysis of long non-coding RNAs (lncRNAs)
Our Team
Meet Our Hardworking Team
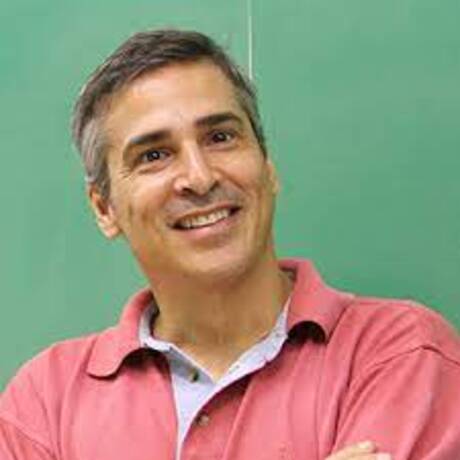
André de Carvalho
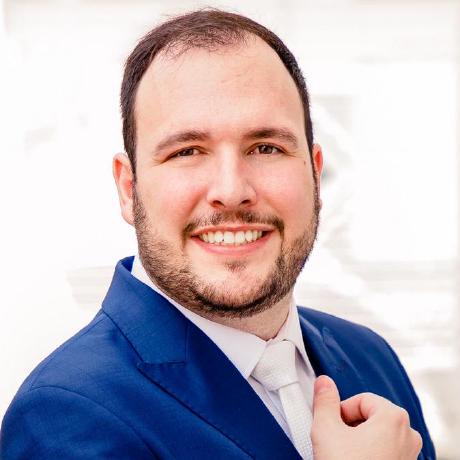
Robson Bonidia
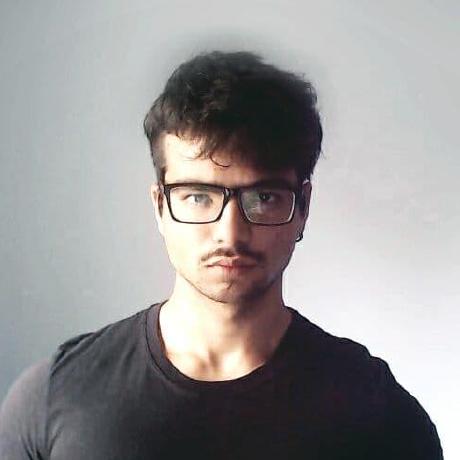
Breno de Almeida
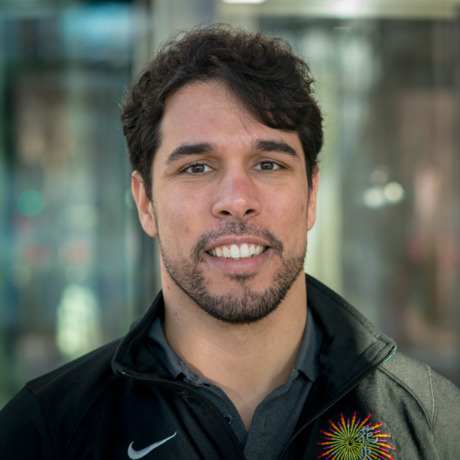
Ulisses da Rocha
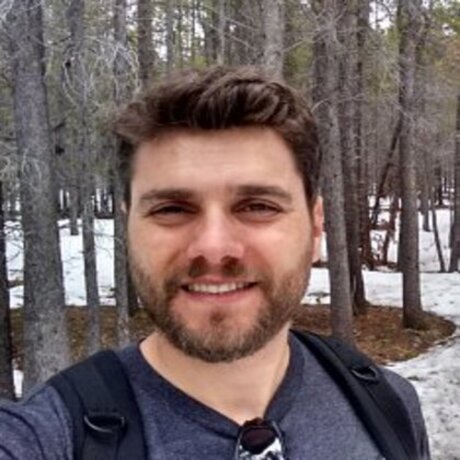
Danilo Sanches
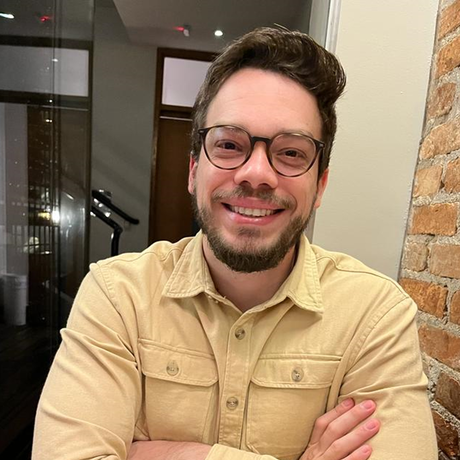
Anderson Santos
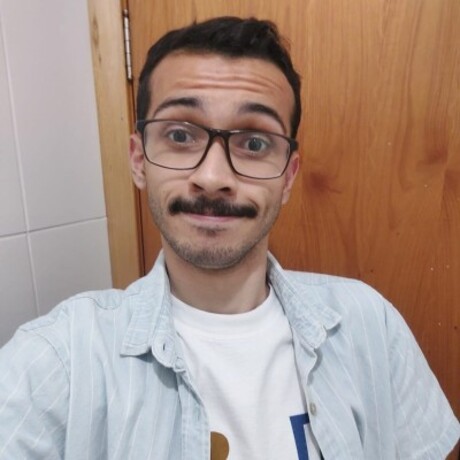
Bruno Florentino
F.A.Q
Frequently Asked Questions
-
Lay summary of the proposed package?
Recent technological advances have allowed an exponential expansion of biological sequence data, and the extraction of meaningful information through Machine Learning (ML) algorithms. This knowledge improved the understanding of the mechanisms related to several fatal diseases, e.g., Cancer and COVID-19, helping to develop innovative solutions, such as CRISPR-based gene editing, coronavirus vaccine, and precision medicine. These advances benefit our society and economy, directly impacting people’s lives in various areas, such as health care, drug discovery, forensic analysis, and food analysis. Nevertheless, ML approaches to biological data require representative, quantitative, and informative features. Necessarily, as many ML algorithms can handle only numerical data, sequences need to be translated into a feature vector. This process, known as feature extraction, is a fundamental step for elaborating high-quality ML-based models in bioinformatics, by allowing the feature engineering stage, with the design and selection of suitable features. Feature engineering, ML algorithm selection, and hyperparameter tuning are often time-consuming processes that require extensive domain knowledge and are performed by a human expert. To deal with this problem, we developed a new package, BioAutoML, which automatically runs an end-to-end ML pipeline. BioAutoML extracts numerical and informative features from biological sequence databases, automating feature selection, recommendation of ML algorithm(s), and tuning of hyperparameters, using Automated ML (AutoML). Our experimental results demonstrate the robustness of our proposal across various domains, such as SARS-CoV-2, anticancer peptides, HIV sequences, and non-coding RNAs. BioAutoML has a high potential to significantly reduce the expertise required to use ML pipelines, aiding researchers in combating diseases, particularly in low- and middle-income countries. This initiative can provide biologists, physicians, epidemiologists, and other stakeholders with an opportunity for widespread use of these techniques to enhance the health and well-being of their communities.
-
Publications
[Link][Conference] BONIDIA, Robson Parmezan; CARVALHO, André Carlos Ponce de Leon Ferreira de. BioAutoML: Democratizing Machine Learning in Life Sciences. In: PRÊMIO ARTUR ZIVIANI - CONCURSO DE TESES E DISSERTAÇÕES (DOUTORADO) - SIMPÓSIO BRASILEIRO DE COMPUTAÇÃO APLICADA À SAÚDE (SBCAS), 24. , 2024, Goiânia/GO. Anais [...]. Porto Alegre: Sociedade Brasileira de Computação, 2024 . p. 85-90. ISSN 2763-8987. [Link][Conference] FLORENTINO, Bruno R.; BONIDIA, Robson P.; CARVALHO, André C. P. L. F. de. Breaking Barriers: Democratizing Machine Learning for RNA-Protein Interaction Prediction in Life Sciences. In: CONCURSO DE TRABALHOS DE INICIAÇÃO CIENTÍFICA - SIMPÓSIO BRASILEIRO DE COMPUTAÇÃO APLICADA À SAÚDE (SBCAS), 24. , 2024, Goiânia/GO. Anais [...]. Porto Alegre: Sociedade Brasileira de Computação, 2024 . p. 7-12. ISSN 2763-8987. [Link][IF 2022: 6.000] Florentino, B. R., Bonidia, R. P., Sanches, N. H., da Rocha, U. N., & de Carvalho, A. C. BioPrediction-RPI: Democratizing the Prediction of Interaction Between Non-Coding RNA and Protein with End-to-End Machine Learning. Computational and Structural Biotechnology Journal, 2024. [Link][IF 2022: 4.100] AVILA SANTOS, ANDERSON P.; DE ALMEIDA, BRENO L. S.; BONIDIA, ROBSON P.; STADLER, PETER F.; STEFANIC, POLONCA; MANDIC-MULEC, INES; ROCHA, ULISSES; SANCHES, DANILO S.; DE CARVALHO, ANDRÉ C.P.L.F. BioDeepfuse: a hybrid deep learning approach with integrated feature extraction techniques for enhanced non-coding RNA classification. Rna Biology, v. 21, p. 1-12, 2024. [Link][Conference] BONIDIA, R. P.; SANTOS, A. P. A.; ALMEIDA, B. L. S.; STADLER, P.; ROCHA, U. N.; SANCHES, D. S.; CARVALHO, A. C. P. L. F. BioAutoML: End-to-End Machine Learning Package for Life Sciences. In: 10th FEMS Congress of European Microbiologists, 2023, Hamburg - Germany. 10th FEMS Congress of European Microbiologists, 2023. [Link][IF 2021: 13.994] BONIDIA, ROBSON P; SANTOS, ANDERSON P AVILA; DE ALMEIDA, BRENO L S; STADLER, PETER F; DA ROCHA, ULISSES N; SANCHES, DANILO S; DE CARVALHO, ANDRÉ C P L F. BioAutoML: automated feature engineering and metalearning to predict noncoding RNAs in bacteria. Briefings in Bioinformatics, v. 1, p. 1-13, 2022. [Link][IF 2021: 2.738] BONIDIA, ROBSON P; SANTOS, ANDERSON P AVILA; DE ALMEIDA, BRENO L S; STADLER, PETER F; DA ROCHA, ULISSES N; SANCHES, DANILO S; DE CARVALHO, ANDRÉ C P L F. Information Theory for Biological Sequence Classification: A Novel Feature Extraction Technique Based on Tsallis Entropy. Entropy, v. 24, p. 1398, 2022. [Link][IF 2021: 13.994] BONIDIA, ROBSON P; DOMINGUES, DOUGLAS S; SANCHES, DANILO S; DE CARVALHO, ANDRÉ C P L F. MathFeature: feature extraction package for DNA, RNA and protein sequences based on mathematical descriptors. Briefings in Bioinformatics, v. 1, p. 1-10, 2022. [Link][IF 2020: 11.622] BONIDIA, ROBSON P; SAMPAIO, LUCAS D H; DOMINGUES, DOUGLAS S; PASCHOAL, ALEXANDRE R; LOPES, FABRÍCIO M; DE CARVALHO, ANDRÉ C P L F; SANCHES, DANILO S. Feature extraction approaches for biological sequences: a comparative study of mathematical features. Briefings in Bioinformatics, v. 00, p. 1-20, 2021. [Link][IF 2019: 3.745] BONIDIA, ROBSON P.; MACHIDA, JAQUELINE SAYURI; NEGRI, TATIANNE C.; ALVES, WONDER A. L.; KASHIWABARA, ANDRE Y.; DOMINGUES, DOUGLAS S.; DE CARVALHO, ANDRE C.P.L.F.; PASCHOAL, ALEXANDRE R.; SANCHES, DANILO S. A Novel Decomposing Model with Evolutionary Algorithms for Feature Selection in Long Non-Coding RNAs. IEEE Access, v. 1, p. 1-15, 2020.
-
Documentations
BioAutoML: Documentation MathFeature: Documentation BioDeepFuse: Documentation